How thousands of helpers are training up our fact checking AI
Last autumn, we asked you to help us in a new way: by taking part in our “Claim Challenge”. We were bowled over by the response, with over four thousand of you helping us match 250,000 claims. But how exactly did all this claim matching make a difference?
Join 72,547 people who trust us to check the facts
Subscribe to get weekly updates on politics, immigration, health and more.
What we asked you do to
Bad information can evolve and spread quickly, even after we’ve found a statement to be wrong–making it much harder to stop it from spreading further and causing more harm.
We're building a claim matching tool that uses AI to identify when a claim (that’s a statement made in the news or on social media) that we’ve checked has been repeated in the media or by a politician.
The first version of our tool learned from only a small set of examples (hashed out by an energy-drink fuelled team Full Fact) and was far from perfect.
We needed lots of helpers to tell us when our tool was accurately matching statements, and when it wasn’t–so that it could better spot repeated instances of false information.
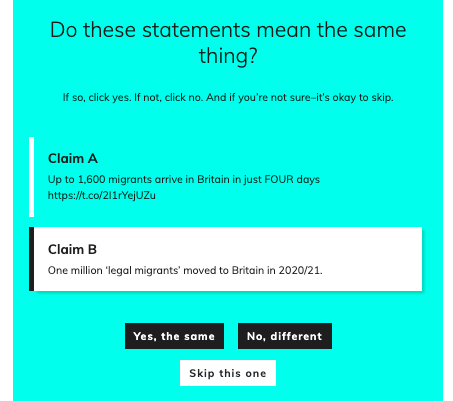
The difference you’ve made
The new model is substantially better than the old, and even though it still makes mistakes it does allow us to find more repeats of misleading claims.
Thanks to your help, we can better spot “semantically similar” phrases.
For example, when the Highway Code was updated recently, there was some confusion about what had changed. Our fact checkers wrote about several changes, including the claim:
Cyclists on either side of a vehicle have priority when cars are turning.
A newspaper writing about the same section of the Code addressed their comments to drivers in this way:
Cyclists can pass you on the left as well as the right when you're in a jam: Motorists need to keep their wits about them on congested routes, as the Highway Code update now says a cyclist is allowed to pass them when in slow-moving or stationary traffic both on the right and the left.
While both sentences explicitly mention "cyclists", the claim we checked refers to "a vehicle" and "cars", while the newspaper article refers to "motorists". This means that a simple search based on specific words would never find this match. Further, while motorists often drive cars the words are clearly not synonyms, showing that the updated system is capable of more nuanced interpretations than a simple dictionary or thesaurus could provide.
Similarly, the claim refers to "either side of a vehicle" which the paper describes as "both on the right and the left". Again, these are phrases that in this context have a similar meaning, but are expressed in quite different words.
Anyone reading this claim might be interested in reading our fact check article to understand the context. The previous version of our Claim Matching tool did not find this article. However, the newer version, built with the help of our supporters, correctly recognised that the fact check article was relevant to this claim, despite differences in the words used.
Note that not every sentence that mentions “cyclist”, “motorist”, “right” and “left” is a good match for the claim, but the more examples we have to train our model on, the better it gets at deciding when each sentence does match a fact check.
Now, we need more help to match statistics
Claim matching is not the only place we use artificial intelligence at Full Fact. We are currently developing a prototype “Stats Checker” tool that is able to verify numerical claims against official statistics, giving our fact checkers vital information as quickly as possible. First, it identifies the key elements of a claim, such as the topic and any relevant dates. It then looks up the corresponding figures from the published statistics and makes a comparison.
However, there are many ways to express even the simplest idea in English. So perhaps not surprisingly, one challenge we’ve found is teaching our AI model how to find the key information from sentences found in newspapers, parliamentary debates and social media.
For example, when shown the sentence
Average pay grew by 3.8% in the quarter to November, while inflation soared to 5.1% in November.
the tool has to determine that the value “3.8%” refers to the topic of “average pay” and that this is an increase (“grew”). It can then verify whether this is true (or at least that it was true when the claim was made). The tool must also recognise the claim that inflation increased (“soared”) by 5.1%. To check if it has interpreted the sentence correctly, the AI tool will ask you questions like:
- Is this a claim about “pay”?
- Does this claim relate “pay” to “3.8%”?
- Does this claim relate “inflation” to “5.1%”?
- In this claim, is “5.1%” the final value?
In each case a simple “yes” or “no” is all that’s required: we’re not asking whether the claim is true or not, only whether the tool has interpreted the text correctly.
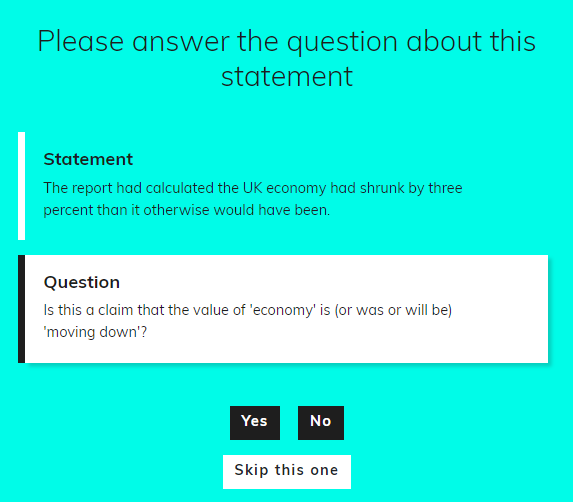
When shown some other sentences, the tool does misinterpret them. For example, when shown
If one in five people who eat beef switched to something else for one weekly meal, that would reduce the carbon footprint by 10 per cent.
the system asks:
- Does this claim relate “five” to “carbon”?
So it has misinterpreted both the number (“five” is part of “one in five”) and the topic (“carbon” is part of “carbon footprint”).
Just like when we are learning, the AI tool needs to know when it is right and when it is wrong
And this is where we’re asking for your help once again! Can you help us train our AI to better interpret language and numbers, so that human fact checkers can do their jobs faster?
The more examples it has, the faster it can learn.
So please, if you want to make a difference for fact checkers around the world, and you have the time, have a go at our next AI challenge.
Blog image credit: betterimagesofai.org